The world of ecommerce is becoming increasingly complex as more and more people begin to buy and sell goods online. The more avenues there are to sell on, the harder it becomes to track all of that data to make an accurate demand forecast. As the channels companies can sell through continue to diversify, the ability to parse through sales data and predict market trends using advanced technologies like automation and artificial intelligence (AI) will quickly become an invaluable resource.
Demand forecasting helps decision-making by informing how many units need to be ordered, preventing companies from overstocking on inventory that won't sell. It is a powerful tool for businesses expanding into new territories, new products, and demand fluctuations. However, many companies still struggle to correctly factor demand into their replenishment process.
When companies have an accurate demand forecast, it becomes much easier for the business to anticipate future cash flow requirements. Often, peak season merchandise must be paid for in an off-peak period when capital is scarce. An accurate forecast can prevent placing undue pressure on a business at an inopportune time.
Keep reading to learn how it all works and what tools and information you need to leverage demand forecasting in your business.
What is demand forecasting?
Demand forecasting, sometimes called sales forecasting, uses historical sales data and predictive analytics to predict future customer demand for products. The primary goal of demand forecasting is to calculate and anticipate what your customer needs and purchase plans will be in the future, considering both quantity and type of items. It's a crucial factor in the business planning process—for everything from marketing strategies to inventory planning to supply chain management.
Why You Should Forecast Demand
Long-term demand forecasting is valuable for nearly any business. Projections for profit margins, expenditure, inventory turnover, risk assessment, and more are often essential for business planners at all levels and across all departments of an organization. Forecasting allows key stakeholders to plan for multiple business functions, including future labor needs, inventory costs, advertisement campaigns, and individual regional sales projections.
Different industries and companies may employ various demand forecasting techniques—including more short-term demand forecasting—based on their available data, the level of accuracy required, and the seasonality of their businesses. This enables businesses to make data-driven business decisions based on forecasted inventory levels, potential sales, and supply chain trends as well as anticipated disruptions.
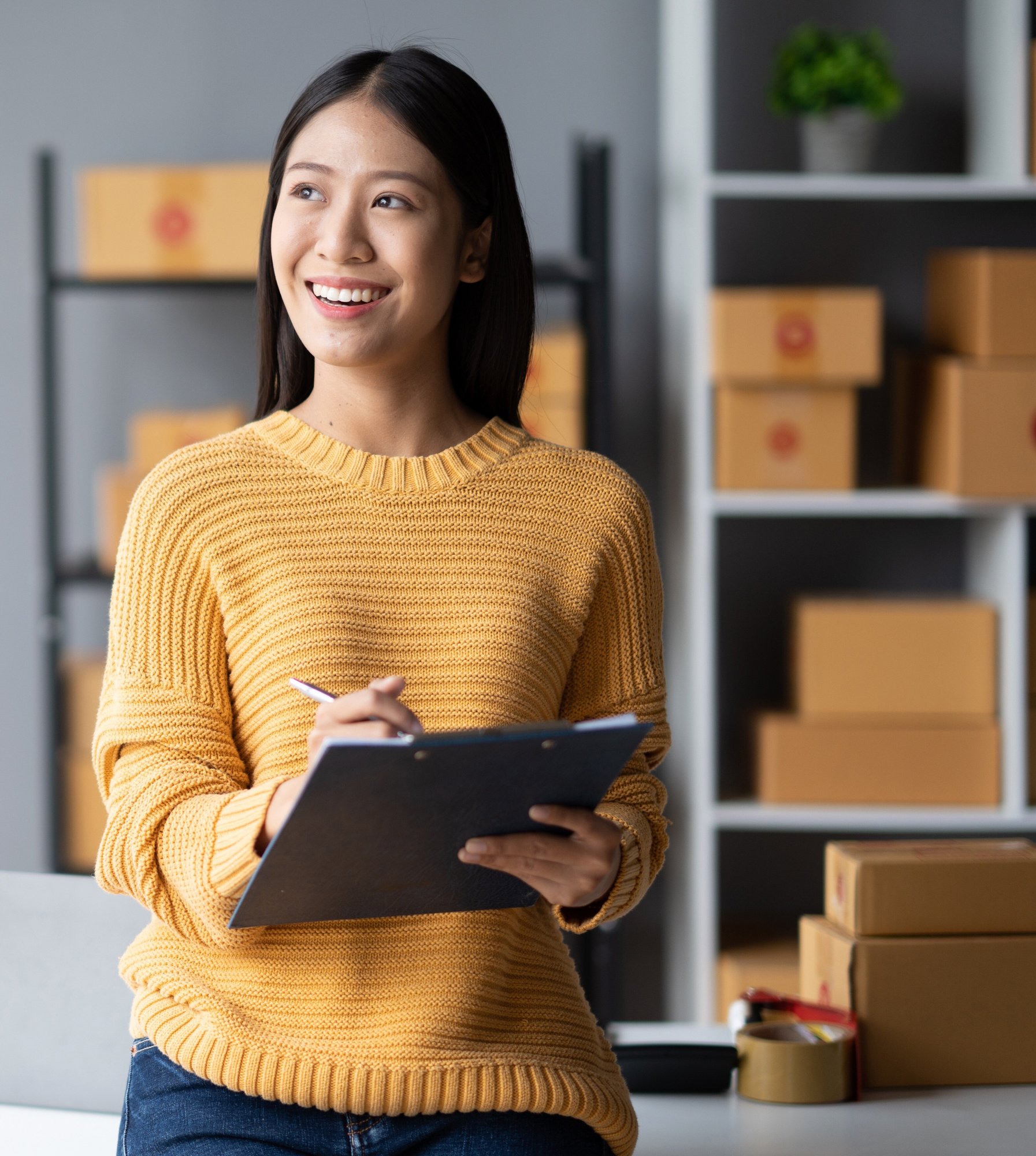
Demand forecasting is particularly useful in retail and ecommerce, where accurate and reliable projections are a critical for improving inventory management. Forecasts can model optimal stock levels for individual products at different times of the year to determine the best times to reorder—so you can avoid stockouts and overstocking—making this a skill worth mastering.
Unsurprisingly, the amount of data required for the demand forecasting process can be overwhelming without dedicated software. Luckily, forecast accuracy improves significantly with the right tools such as order management systems (OMS) and inventory management software. With an integrated ecommerce and/or fulfillment technology stack, you can track in-depth inventory information like stock levels and actual sales velocity for each SKU, allowing you to plan for the future and allocate the right resources to the right channels and warehouses.
Of course, gathering your data is only half the battle. As McKinsey points out, you must also act on that data by incorporating it into your business model, meaning you’ll need to foster a data-driven culture within your organization. If demand forecasting is new to you, brainstorm among your staff how best to use the data you’ve gained from your research. Staff training or hiring a consultant can pay dividends when first implementing demand forecasting, though sometimes it's as simple as carefully strategizing among your internal staff.
6 Demand Forecasting Methods
Demand forecasting, sometimes called sales forecasting, uses historical sales data and predictive analytics to predict future customer demand for products. The primary goal of demand forecasting is to calculate and anticipate what your customer needs and purchase plans will be in the future, considering both quantity and type of items. It's a crucial factor in the business planning process—for everything from marketing strategies to inventory planning to supply chain management.
1. Collective Opinion
The collective opinion method of data forecasting leverages the knowledge and experience of a company’s sales team to aggregate data on customer demand. Members of the sales team report on the sales performance of individual products in their respective regions, allowing you to take a broader view of overall demand from granular data.
Factors like product prices, related marketing campaigns, employment opportunities, customer economic data, and competitors are all considered. It's important to remember that demand forecasting relies on both the team's judgment and the data, so use a systematic approach for gathering and prioritizing this information to reduce subjectivity.
If you look at sales data from an established base demand, you can compare highs, lows, peaks, and seasonal trends. Inventory management software tends to have built-in features allowing sales executives to gather and analyze this data to perfect their seasonal inventory strategies.
2. Customer Surveys
Customer surveys can provide key information on customer satisfaction, expectations, desires, and needs. This form of market research also examines individual customer demographics and economic data to better understand the customer base.
There are many ways you can conduct these surveys:
- Sample Survey: A sample of potential buyers are scientifically selected and interviewed to determine their buying habits.
- Complete Enumeration Survey: To gather a more expansive data set, the largest sample of potential buyers possible is targeted for interviews.
- End-Use Survey: This survey gathers data from companies in related industries to determine their view on end-use demand.
3. Barometric Method
The barometric method involves using economic indicators to predict trends. All of these indicators can be used to measure current, past, and future activity when it comes to your business, allowing for better inventory management, supply chain management, and demand planning.
- Leading Indicator: A performance indicator that might predict future events.
- Lagging Indicator: An indicator of past performance that analyzes the impact of past events.
- Coincidental Indicator: A measure of current events happening simultaneously in real time or close together over a short time frame.
There are many ways we can use these indicators to our advantage. For example, an increase in customer complaints is a leading indicator of emerging problems in production, distribution, or customer service. Meanwhile, a sharp spike in sales might be a leading indicator that business is picking up. The spike could be an anomaly or the beginning of a trend—businesses can use lagging indicators like growth and retention to provide more context. Finally, a good example of a coincidental indicator would be inventory turnover, which can be monitored in real time to demonstrate current sales activity.
4. Expert Opinion
To determine future activity, you can seek expert advice from external contractors. Market experts use different methods, such as the Delphi method, which involves a series of questionnaires designed to gather information needed to make predictions. Based on the findings and consultations with the relevant experts, you’ll be able to create a better roadmap for what your business can expect in the coming weeks, months, or even years. This method can be cost-effective and time-efficient, allowing companies to implement it quickly.
5. Market Experimentation
Market experiments can be carried out under controlled conditions to inform retailers of consumer behavior. A/B testing of different discounts, special offers, site features/layout/design elements, and messaging can inform a company of what appeals to customers.
6. Statistical Method
Using statistics can also be very informative when it comes to demand forecasting. Statistical methods are detailed, reliable, and often cost-effective. Some versions of statistical methods include:
- Regression Analysis: This method allows companies to identify and analyze the relationships between different variables including sales, conversions, email signups, and more. Considering how each is affected by the other can help a company allocate resources to the right area and boost sales.
- Trend Projection: This method relies on larger data sets of past sales to establish performance history over time, identify trends, and extrapolate potential future trends. This is also a useful tool for more effective inventory management at different times of the year.
Selecting Demand Forecasting Software in 3 Steps
Many tools and software programs facilitate demand forecasting. To find the right solution for your business, consider your needs, the specific capabilities that will help you achieve your goals, and whether you have the appropriate internal resources for demand forecasting.
Step 1: Identify Your Demand Planning Requirements
Take time to carefully document your sales and demand trends in a detailed specification document. Consider the past and current state of your inventory management and supply chain management: what were some recent issues, and what do you need to mitigate them in the future? Regarding future performance, are there areas of your business that you feel you lack insight into? What constraints in terms of budget and project lifecycle might emerge when considering the right tools for forecasting
Step 2: Find the Most Capable Software
Once you know which areas you want to focus on, consider the software features you’ll need to facilitate your demand planning. Some common features include:
- Hypothetical/complex situation modeling
- Price modeling
- Support for granular metrics like econometric and cluster analysis
- Performance measurements to gauge the value & accuracy of forecasts
- Integration capabilities and ease of use
- Multi-tier planning for separate outlets, regions, or franchises
- Artificial intelligence & machine learning (ML) capabilities
Choosing the right ecommerce operations software is an important decision that can make a massive difference in how you optimize your inventory, manage your cash flow, plan for the future, and (ideally) grow your bottom line. Your best bet is to create a team of internal subject matter experts and power users to participate in selecting any new demand forecasting solution or creating new procedures. This will help your team adopt the new solution faster and manage internal change and expectations.
Step 3: Involve Your Team
To get the best results, ensure your staff has the training, knowledge, and technical capabilities to not only use your demand forecasting software but also know why they should use it. Budgeting for a short-term decline in productivity while your team undergoes training will help ensure long-term adoption and success while maximizing the benefits of the new solution.
If you're using multiple, separate tools to meet for demand planning, you may need certain staff with specific technical skills to keep all your systems in sync. You’ll also want to keep an eye on how accurately your team can share, merge, or compare data from different systems. Alternatively, you may find that a standalone, all-in-one solution meets your business needs better than a series of individual tools.
How to Improve Your Demand Forecasting Strategy
Clearly, the demand forecasting process is a lot more scientific than gazing into a crystal ball, but it is often a mixture of art and science, which means it won’t always be perfect. There is no one-size-fits-all demand forecasting model—finding the right models and methods for your business can be a matter of trial and error. Below are some proven ways to improve your demand forecasting strategy:

Foster Internal Partnerships
Often within businesses, silos can be created because everyone is focused on their area rather than the business as a whole. In a sales-focused organization, this can create large gaps and missteps when reviewed across the business. But the initiatives of one department often affect others; for instance, when stores or sales channels are closed, renovated, or opened in new markets, this will significantly impact a demand forecast.
Imagine your business decided to open an Instagram store or other social commerce outlet within the next month. Would your operations team know well enough in advance to have enough inventory available to ensure the success of this channel?
Keeping all departments on the same page is critical. For example, fostering a partnership between marketing and inventory teams ensures that the products included in key advertising campaigns, social media content, or email marketing have enough volume in stock to satisfy boosted customer demand. Publishing a marketing calendar that outlines key moments in your go-to-market strategy with company-wide visibility is an easy way to strengthen your organizational communication.

Pay Attention to Intermittent Demand
It's common to see ecommerce retailers selling camping gear, which experiences intermittent demand, at a reduced rate during the winter to compensate for an over-anticipation of future sales during the sunnier months. This miscalculation results in an inventory surplus because seasonal demand wasn't properly calculated.
Intermittent demand forecasting relates to predicting sales for a product with sporadic sales. Sometimes, the data on these products shows gaps in sales that relate to supply chain management or common sales trends; other times, the patterns are more random and difficult to predict. In these cases, traditional demand forecasting methods do not always apply and can lead to errors. Some common pitfalls and forecasting errors that companies might run into when forecasting intermittent demand include:
-
-
- Failing to account for seasonal demand
- Using identical forecasting calculations for products with different sales behavior
- Failing to filter out promotional sales activity that can skew actual demand trends
- Not aligning the demand forecast to the planned marketing calendar or, conversely, not aligning the marketing calendar to the available-to-sell inventory
- Overestimating the quantity of products available to sell (for example, if skilled labor or equipment is required to make personalized products but is limited)
-

Be Flexible and Proactive
Even with a forecast that is completely informed by all available data and the most sophisticated and accurate solutions, there is still a chance that you could miscalculate sales forecasts—especially during peak sales seasons or viral demand surges (frequently fueled by social media). The last thing you want is to miss out on customer demand.
Proactively preparing for this by implementing dropshipping options or the ability to share inventory across sales channels by deploying modern, flexible solutions like Extensiv could allow your business to reduce overall buy quantities while not missing out on any sales opportunities.
Additionally, using the right inventory restocking model can prevent under- or overstocking when your demand forecasting accuracy isn’t 100%. Based on trade-offs between time, revenue, and profit, retailers use several different restocking models to meet their goals:
-
-
- Continuous Inventory System: This model constantly and automatically restocks inventory below a certain cutoff point, regardless of other external factors.
- Periodic Inventory System: This system requires that a retailer periodically assess the stock levels during a given time series and decide how much stock is needed based on current demand.
- Economic Order Quantity (EOQ): This model calculates the number of SKUs a retailer should restock to minimize costs and maximize value.
-
Elevate Your Demand Forecasting with Extensiv
Improving your demand forecasting is a proven way to increase profits, mitigate unnecessary costs, and optimize your supply chain—especially when you have the right tools. Extensiv’s complete solutions for brands can bring your ecommerce business to the next level.
Extensiv’s cloud-based software for retailers, ecommerce merchants, and omnichannel brands provides robust, end-to-end data analytics and reporting features along with demand planning capabilities to improve your inventory flow and work smarter, not harder. Using historical sales data, you can calculate how many units you need to reorder within a certain time period and the exact date a purchase order should be issued—and you can even factor in projected growth to these calculations on a SKU-by-SKU basis. And that’s not the end; our order management system then automatically creates purchase orders with recommended reorder quantities, streamlining your replenishment process tailored to your precise demand forecasts.
Want to learn more about Extensiv’s platform? Request a demo today!
-
You’ll read about:
Be the first to know
Subscribe to our newsletter